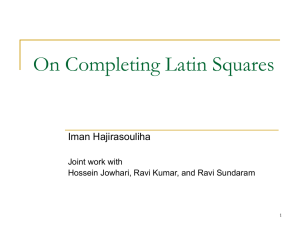
Precondition Control and the Progression Algorithm
... advantage which has indeed been verified empirically in experiments and in the AIPS planning competitions. Planners using search control have shown several orders of magnitude superior performance in multiple planning domains. In many of these domains, problems of size formerly beyond the capabiliti ...
... advantage which has indeed been verified empirically in experiments and in the AIPS planning competitions. Planners using search control have shown several orders of magnitude superior performance in multiple planning domains. In many of these domains, problems of size formerly beyond the capabiliti ...
Range-Efficient Counting of Distinct Elements in a Massive Data
... 1.1. Our results. We consider the problem of range-efficient computation of F0 , defined as follows. The input stream is R = r1 , r2 , . . . , rm , where each stream element ri = [xi , yi ] ⊂ [1, n] is an interval of integers xi , xi + 1, . . . , yi . The length of an interval ri could be any number be ...
... 1.1. Our results. We consider the problem of range-efficient computation of F0 , defined as follows. The input stream is R = r1 , r2 , . . . , rm , where each stream element ri = [xi , yi ] ⊂ [1, n] is an interval of integers xi , xi + 1, . . . , yi . The length of an interval ri could be any number be ...
The Laws of Probability and the Law of the Land
... ,1Cohen's arguments as to the difficulty of quantifying the burden of persuasion in civil cases are considered in more detail in Kaye, The Paradox of the Gatecrasherand Other Stories, 1979 ARIz. ST. L.J. 101. The analysis in Lempert, supra note 6, implicitly helps resolve some of the other "anomalie ...
... ,1Cohen's arguments as to the difficulty of quantifying the burden of persuasion in civil cases are considered in more detail in Kaye, The Paradox of the Gatecrasherand Other Stories, 1979 ARIz. ST. L.J. 101. The analysis in Lempert, supra note 6, implicitly helps resolve some of the other "anomalie ...
Kernel Estimation and Model Combination in A Bandit Problem with
... Different variants of the bandit problem motivated by real applications have been studied extensively in the past decade. One promising setting is to assume that the reward distribution of each bandit arm is associated with some common external covariate. More specifically, for an l-armed bandit pro ...
... Different variants of the bandit problem motivated by real applications have been studied extensively in the past decade. One promising setting is to assume that the reward distribution of each bandit arm is associated with some common external covariate. More specifically, for an l-armed bandit pro ...
On Lattices, Learning with Errors, Random Linear Codes, and
... best known polynomial time algorithms for them yield only mildly subexponential approximation factors [24, 38, 5]. It is conjectured that there is no classical (i.e., non-quantum) polynomial time algorithm that approximates them to within any polynomial factor. Lattice-based constructions of one-way ...
... best known polynomial time algorithms for them yield only mildly subexponential approximation factors [24, 38, 5]. It is conjectured that there is no classical (i.e., non-quantum) polynomial time algorithm that approximates them to within any polynomial factor. Lattice-based constructions of one-way ...
Simulated annealing

Simulated annealing (SA) is a generic probabilistic metaheuristic for the global optimization problem of locating a good approximation to the global optimum of a given function in a large search space. It is often used when the search space is discrete (e.g., all tours that visit a given set of cities). For certain problems, simulated annealing may be more efficient than exhaustive enumeration — provided that the goal is merely to find an acceptably good solution in a fixed amount of time, rather than the best possible solution.The name and inspiration come from annealing in metallurgy, a technique involving heating and controlled cooling of a material to increase the size of its crystals and reduce their defects. Both are attributes of the material that depend on its thermodynamic free energy. Heating and cooling the material affects both the temperature and the thermodynamic free energy. While the same amount of cooling brings the same amount of decrease in temperature it will bring a bigger or smaller decrease in the thermodynamic free energy depending on the rate that it occurs, with a slower rate producing a bigger decrease.This notion of slow cooling is implemented in the Simulated Annealing algorithm as a slow decrease in the probability of accepting worse solutions as it explores the solution space. Accepting worse solutions is a fundamental property of metaheuristics because it allows for a more extensive search for the optimal solution.The method was independently described by Scott Kirkpatrick, C. Daniel Gelatt and Mario P. Vecchi in 1983, and by Vlado Černý in 1985. The method is an adaptation of the Metropolis–Hastings algorithm, a Monte Carlo method to generate sample states of a thermodynamic system, invented by M.N. Rosenbluth and published in a paper by N. Metropolis et al. in 1953.