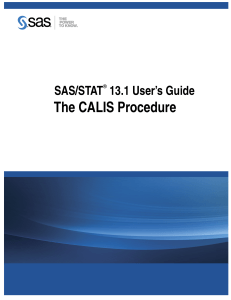
An introduction to Bootstrap Methods Outline Monte Carlo
... easy to construct a bootstrap test. Sampling from the null hypothesis is not so easy because we need to transform F̂n to reflect the null hypothesis! Therefore, permutation tests are complementary to the bootstrap tests. ...
... easy to construct a bootstrap test. Sampling from the null hypothesis is not so easy because we need to transform F̂n to reflect the null hypothesis! Therefore, permutation tests are complementary to the bootstrap tests. ...
Applied Missing Data Analysis
... analysis examples. The level with which to integrate specific software programs was an issue that presented me with a dilemma throughout the writing process. In the end, I chose to make the analysis examples independent of any program. In the 2 years that it took to write this book, software program ...
... analysis examples. The level with which to integrate specific software programs was an issue that presented me with a dilemma throughout the writing process. In the end, I chose to make the analysis examples independent of any program. In the 2 years that it took to write this book, software program ...
Méthodes non-paramétriques pour la prévision d - ENAC
... that detect and solve conflicts between trajectories. In this scope, this work proposes two non-parametric interval prediction methods in the regression context. These methods are designed to predict intervals that contain at least a desired proportion of the conditional distribution of the response ...
... that detect and solve conflicts between trajectories. In this scope, this work proposes two non-parametric interval prediction methods in the regression context. These methods are designed to predict intervals that contain at least a desired proportion of the conditional distribution of the response ...
Statistics 1: MATH11400
... Mathematical statistics and data analysis by JA Rice This covers both Probability and Statistics material and some of the second year Statistics unit. It combines modern ideas of data analysis, using graphical and computational techniques, with more traditional approaches to mathematical statistics. ...
... Mathematical statistics and data analysis by JA Rice This covers both Probability and Statistics material and some of the second year Statistics unit. It combines modern ideas of data analysis, using graphical and computational techniques, with more traditional approaches to mathematical statistics. ...
Syllabus 2008 - Institute of Cost Accountants of India
... A purse contains 1 rupee coin, 50 paisa coin, 25 paisa coin. The ratio of their numbers are x : y : z . The ratio of their values: (a) 4x: 2y : z (b) 2x : 3y : z (c) 4x: 3y : z (d)x : 2y : 4z Of the four numbers in proportion, if the product of two middle numbers is 48, the other numbers are : a) 32 ...
... A purse contains 1 rupee coin, 50 paisa coin, 25 paisa coin. The ratio of their numbers are x : y : z . The ratio of their values: (a) 4x: 2y : z (b) 2x : 3y : z (c) 4x: 3y : z (d)x : 2y : 4z Of the four numbers in proportion, if the product of two middle numbers is 48, the other numbers are : a) 32 ...
Coefficient of determination
In statistics, the coefficient of determination, denoted R2 or r2 and pronounced R squared, is a number that indicates how well data fit a statistical model – sometimes simply a line or a curve. An R2 of 1 indicates that the regression line perfectly fits the data, while an R2 of 0 indicates that the line does not fit the data at all. This latter can be because the data is utterly non-linear, or because it is random.It is a statistic used in the context of statistical models whose main purpose is either the prediction of future outcomes or the testing of hypotheses, on the basis of other related information. It provides a measure of how well observed outcomes are replicated by the model, as the proportion of total variation of outcomes explained by the model (pp. 187, 287).There are several definitions of R2 that are only sometimes equivalent. One class of such cases includes that of simple linear regression where r2 is used instead of R2. In this case, if an intercept is included, then r2 is simply the square of the sample correlation coefficient (i.e., r) between the outcomes and their predicted values. If additional explanators are included, R2 is the square of the coefficient of multiple correlation. In both such cases, the coefficient of determination ranges from 0 to 1.Important cases where the computational definition of R2 can yield negative values, depending on the definition used, arise where the predictions that are being compared to the corresponding outcomes have not been derived from a model-fitting procedure using those data, and where linear regression is conducted without including an intercept. Additionally, negative values of R2 may occur when fitting non-linear functions to data. In cases where negative values arise, the mean of the data provides a better fit to the outcomes than do the fitted function values, according to this particular criterion.