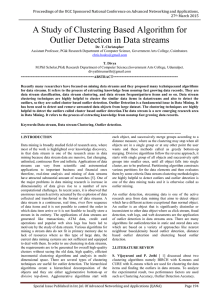
A Study of Clustering Based Algorithm for Outlier Detection in Data
... distance measure, where as the clustering may stop when all Data mining is broadly studied field of research area, where objects are in a single group or at any other point the user most of the work is highlighted over knowledge discovery, wants and these methods called as greedy bottom-up in that d ...
... distance measure, where as the clustering may stop when all Data mining is broadly studied field of research area, where objects are in a single group or at any other point the user most of the work is highlighted over knowledge discovery, wants and these methods called as greedy bottom-up in that d ...
An Efficient Algorithm for Mining Frequent Items in Data Streams
... technique called as dynamic tree restructuring technique to handle the stream data. The main disadvantage of this algorithm is every time a new item arrives, it reconstructs the tree. So it causes more memory space as well as time. Pauray S.M. Tsai [13] proposed a new technique called the weighted s ...
... technique called as dynamic tree restructuring technique to handle the stream data. The main disadvantage of this algorithm is every time a new item arrives, it reconstructs the tree. So it causes more memory space as well as time. Pauray S.M. Tsai [13] proposed a new technique called the weighted s ...
Change-Point Detection in Time-Series Data by Direct Density
... on novelty detection [25, 26], maximum-likelihood estimation [13], and online learning of autoregressive models [34, 33]. Another line of research is based on analysis of subspaces in which time-series sequences are constrained [27, 16, 22]. This approach has a strong connection with a system identi ...
... on novelty detection [25, 26], maximum-likelihood estimation [13], and online learning of autoregressive models [34, 33]. Another line of research is based on analysis of subspaces in which time-series sequences are constrained [27, 16, 22]. This approach has a strong connection with a system identi ...
Outlier Ensembles - Outlier Definition, Detection, and Description
... of models (eg. decision trees, rules, Bayes) or many instantiations of the same class, and vote on the class label of a test instance. • Bagging: Sample repeatedly from training data, and vote on the class label of a test instance. • Boosting; Sequentially select more “difficult” subsets of the traini ...
... of models (eg. decision trees, rules, Bayes) or many instantiations of the same class, and vote on the class label of a test instance. • Bagging: Sample repeatedly from training data, and vote on the class label of a test instance. • Boosting; Sequentially select more “difficult” subsets of the traini ...
Comparative Study of Quality Measures of Sequential Rules for the
... therefore can give the better classes. In fact, the algorithms need to run multiple times with different initial states to obtain a better outcome by following each iteration the reallocation mechanism that reallocate points between classes. Each initialization (set number of clusters) corresponds t ...
... therefore can give the better classes. In fact, the algorithms need to run multiple times with different initial states to obtain a better outcome by following each iteration the reallocation mechanism that reallocate points between classes. Each initialization (set number of clusters) corresponds t ...
WHAT IS AN ALGORITHM?
... and zeros, which make up a binary code. A sample instruction might be 100111000 01100110 Second-generation language- These are also called assembly languages and are characterized by abbreviated words, called mnemonics. A sample code might be ‘Add 12,8’ ...
... and zeros, which make up a binary code. A sample instruction might be 100111000 01100110 Second-generation language- These are also called assembly languages and are characterized by abbreviated words, called mnemonics. A sample code might be ‘Add 12,8’ ...
ppt - MIS
... 4. (20 points) Principle components is used for dimensionality reduction then may be followed by cluster analysis – say for segmentation purposes – Consider a two continuous variable problem. Using scatter plots a) Generate a data set where PCA reduces the dimensionality from two to one b) Generate ...
... 4. (20 points) Principle components is used for dimensionality reduction then may be followed by cluster analysis – say for segmentation purposes – Consider a two continuous variable problem. Using scatter plots a) Generate a data set where PCA reduces the dimensionality from two to one b) Generate ...
04Matrix_Classification_1
... • Examples are partitioned recursively based on selected attributes • Test attributes are selected on the basis of a heuristic or statistical measure (e.g., information gain) • Conditions for stopping partitioning • All samples for a given node belong to the same class • There are no remaining attri ...
... • Examples are partitioned recursively based on selected attributes • Test attributes are selected on the basis of a heuristic or statistical measure (e.g., information gain) • Conditions for stopping partitioning • All samples for a given node belong to the same class • There are no remaining attri ...
Learning when everybody knows a bit of something
... (1998), fall in this area, while more recently Snow et al. (2008) showed that employing multiple non-expert annotators can be as effective as employing one expert annotator when building a classifier. Very recently, the interest has shifted towards more directly building classifiers from multi-label ...
... (1998), fall in this area, while more recently Snow et al. (2008) showed that employing multiple non-expert annotators can be as effective as employing one expert annotator when building a classifier. Very recently, the interest has shifted towards more directly building classifiers from multi-label ...
New Outlier Detection Method Based on Fuzzy Clustering
... to multidimensional data, and the ability to model uncertainty within the data. FCM partitions a collection of n data points xi, i = 1, …, n into c fuzzy groups, and finds a cluster center in each group so that the objective function of the dissimilarity measure is minimized. FCM employs fuzzy parti ...
... to multidimensional data, and the ability to model uncertainty within the data. FCM partitions a collection of n data points xi, i = 1, …, n into c fuzzy groups, and finds a cluster center in each group so that the objective function of the dissimilarity measure is minimized. FCM employs fuzzy parti ...
Modeling annotator expertise: Learning when
... (1998), fall in this area, while more recently Snow et al. (2008) showed that employing multiple non-expert annotators can be as effective as employing one expert annotator when building a classifier. Very recently, the interest has shifted towards more directly building classifiers from multi-label ...
... (1998), fall in this area, while more recently Snow et al. (2008) showed that employing multiple non-expert annotators can be as effective as employing one expert annotator when building a classifier. Very recently, the interest has shifted towards more directly building classifiers from multi-label ...
A Data Mining of Supervised learning Approach based on K
... A diversity of application fields include a massive number of datasets. Each dataset consists of a number of variables (features). One of these variables that is considered as a dependent variable (target variable) and is used for prediction in data mining of the supervised learning task. Data minin ...
... A diversity of application fields include a massive number of datasets. Each dataset consists of a number of variables (features). One of these variables that is considered as a dependent variable (target variable) and is used for prediction in data mining of the supervised learning task. Data minin ...
DBSCAN (Density Based Clustering Method with
... Abstract: Data mining has suit an important in research area because of its ability to get valuable information from the data. The data mining uses various clustering algorithms for grouping related objects. One of the most important clustering algorithm is density based clustering algorithm, which ...
... Abstract: Data mining has suit an important in research area because of its ability to get valuable information from the data. The data mining uses various clustering algorithms for grouping related objects. One of the most important clustering algorithm is density based clustering algorithm, which ...
Supervised model-based visualization of high
... Traditionally, similarity is defined in terms of some standard geometric distance measure, such as the Euclidean distance. However, such distances do not generally properly reflect the properties of complex problem domains, where the data typically is not coded in a geometric or spatial form. In thi ...
... Traditionally, similarity is defined in terms of some standard geometric distance measure, such as the Euclidean distance. However, such distances do not generally properly reflect the properties of complex problem domains, where the data typically is not coded in a geometric or spatial form. In thi ...
K-nearest neighbors algorithm
In pattern recognition, the k-Nearest Neighbors algorithm (or k-NN for short) is a non-parametric method used for classification and regression. In both cases, the input consists of the k closest training examples in the feature space. The output depends on whether k-NN is used for classification or regression: In k-NN classification, the output is a class membership. An object is classified by a majority vote of its neighbors, with the object being assigned to the class most common among its k nearest neighbors (k is a positive integer, typically small). If k = 1, then the object is simply assigned to the class of that single nearest neighbor. In k-NN regression, the output is the property value for the object. This value is the average of the values of its k nearest neighbors.k-NN is a type of instance-based learning, or lazy learning, where the function is only approximated locally and all computation is deferred until classification. The k-NN algorithm is among the simplest of all machine learning algorithms.Both for classification and regression, it can be useful to assign weight to the contributions of the neighbors, so that the nearer neighbors contribute more to the average than the more distant ones. For example, a common weighting scheme consists in giving each neighbor a weight of 1/d, where d is the distance to the neighbor.The neighbors are taken from a set of objects for which the class (for k-NN classification) or the object property value (for k-NN regression) is known. This can be thought of as the training set for the algorithm, though no explicit training step is required.A shortcoming of the k-NN algorithm is that it is sensitive to the local structure of the data. The algorithm has nothing to do with and is not to be confused with k-means, another popular machine learning technique.