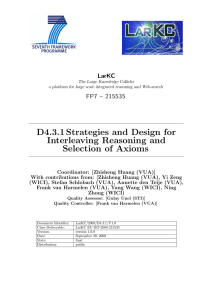
Planning graph as the basis for deriving heuristics for plan synthesis
... Prec(a) and p ∈ Add(a). This computation precludes from consideration potential mutexes r, s, where r and s are not in the add, precondition or delete lists of any single action. As we shall see below, this turns out to be an important limitation in several domains. The sum mutex heuristic used by ...
... Prec(a) and p ∈ Add(a). This computation precludes from consideration potential mutexes r, s, where r and s are not in the add, precondition or delete lists of any single action. As we shall see below, this turns out to be an important limitation in several domains. The sum mutex heuristic used by ...
Planning with Markov Decision Processes
... Markov Decision Processes (MDPs) are widely popular in Artificial Intelligence for modeling sequential decision-making scenarios with probabilistic dynamics. They are the framework of choice when designing an intelligent agent that needs to act for long periods of time in an environment where its ac ...
... Markov Decision Processes (MDPs) are widely popular in Artificial Intelligence for modeling sequential decision-making scenarios with probabilistic dynamics. They are the framework of choice when designing an intelligent agent that needs to act for long periods of time in an environment where its ac ...
Speeding Up Problem-Solving by Abstraction: A Graph
... generality of the results. The graphs used in our experiments are described in Appendix A. Three of these (Bitnet, KL-2000, and Permute7) are drawn from real applications. One (Words) is a real graph that is not associated with any particular application, and three (5-puzzle, Blocks-6, and TOH-7) a ...
... generality of the results. The graphs used in our experiments are described in Appendix A. Three of these (Bitnet, KL-2000, and Permute7) are drawn from real applications. One (Words) is a real graph that is not associated with any particular application, and three (5-puzzle, Blocks-6, and TOH-7) a ...
Cooperative Heuristic Search with Software Agents - Aalto
... noncooperative, explicitly randomized A∗ variant in the standard n-puzzle sliding tile problem context. The results indicate that A! performance increases with the addition of more agents, but that the returns are diminishing. A! is observed to be sensitive to heuristic improvement, but also constra ...
... noncooperative, explicitly randomized A∗ variant in the standard n-puzzle sliding tile problem context. The results indicate that A! performance increases with the addition of more agents, but that the returns are diminishing. A! is observed to be sensitive to heuristic improvement, but also constra ...
Artificial Intelligence Search Algorithms In Travel Planning
... very much connected to problem solving. AI has investigated search methods that allow one to solve path planning problems in large domains. Having formulated problems we need to solve them and it is done by searching through the state space during this process search tree is generated by taking init ...
... very much connected to problem solving. AI has investigated search methods that allow one to solve path planning problems in large domains. Having formulated problems we need to solve them and it is done by searching through the state space during this process search tree is generated by taking init ...
Conceptual Graphs and First Order Logic
... (which are called witnesses in logic) [14]. The witnesses are substituted to all existential quantifiers in the translation of the conceptual graph which must be derived; more precisely, a witness for each occurrence of an existential quantified concept in the graph. The graph to be derived (accordi ...
... (which are called witnesses in logic) [14]. The witnesses are substituted to all existential quantifiers in the translation of the conceptual graph which must be derived; more precisely, a witness for each occurrence of an existential quantified concept in the graph. The graph to be derived (accordi ...
Suggested Solutions to Assignment 3
... Firm 2 do to make its threat credible? If firm 2 threatens to play “aggressive” if Firm 1 plays “aggressive”, it will NOT be credible to Firm 1. Because Firm 1 knows that if it plays “aggressive” in period 1, Firm 2’s best response in period 2 will be “passive”. Because 10 > 9 . Firm 2’s problem is ...
... Firm 2 do to make its threat credible? If firm 2 threatens to play “aggressive” if Firm 1 plays “aggressive”, it will NOT be credible to Firm 1. Because Firm 1 knows that if it plays “aggressive” in period 1, Firm 2’s best response in period 2 will be “passive”. Because 10 > 9 . Firm 2’s problem is ...
A Functional Programming Approach to AI Search Algorithms
... in a list containing only 2-element lists with all the possible layouts of two queens in two columns. This is repeated until col reaches N, when the resulting list will contain all possible solutions. Of course, we could also have written here the recursive breadth-first search in C. The reason we c ...
... in a list containing only 2-element lists with all the possible layouts of two queens in two columns. This is repeated until col reaches N, when the resulting list will contain all possible solutions. Of course, we could also have written here the recursive breadth-first search in C. The reason we c ...
Real-Time Search for Autonomous Agents and
... 3. Controlling learning processes An important capability of real-time search is learning, that is, as in LRTA*, the solution path converges to an optimal path by repeating problem solving trials. In this section, we will focus not on the performance of the first problem solving trial, but on the le ...
... 3. Controlling learning processes An important capability of real-time search is learning, that is, as in LRTA*, the solution path converges to an optimal path by repeating problem solving trials. In this section, we will focus not on the performance of the first problem solving trial, but on the le ...