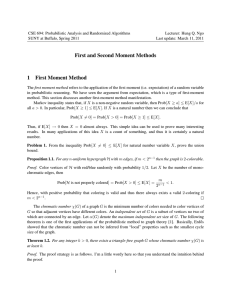
First and Second Moment Methods 1 First Moment Method
... Each graph in the distribution G(n, p) has n vertices, and there is an edge between any pair of vertices with probability p. This is called the Erdős-Rényi model of random graphs. Often we would like to know if a random graph has some property. For example, if the (random) graph represents a P2P n ...
... Each graph in the distribution G(n, p) has n vertices, and there is an edge between any pair of vertices with probability p. This is called the Erdős-Rényi model of random graphs. Often we would like to know if a random graph has some property. For example, if the (random) graph represents a P2P n ...
Efficient Fully-Simulatable Oblivious Transfer∗
... also efficient (without using random oracles). Despite this, recently there has been some progress in this area. In [6], fully simulatable constructions are presented. However, these rely on strong and relatively non-standard assumptions (q-power DDH and q-strong Diffie-Hellman). Following this, pro ...
... also efficient (without using random oracles). Despite this, recently there has been some progress in this area. In [6], fully simulatable constructions are presented. However, these rely on strong and relatively non-standard assumptions (q-power DDH and q-strong Diffie-Hellman). Following this, pro ...
Solve Simple Linear Equation using Evolutionary Algorithm
... optimum are processed in parallel. The calculations required for this feat are obviously much more extensive than for a simple random search [3,9]. ...
... optimum are processed in parallel. The calculations required for this feat are obviously much more extensive than for a simple random search [3,9]. ...
On Worst-Case to Average-Case Reductions for NP Problems
... according to the same distribution (that depends only on the length of the input), then the existence of such a reduction implies that the polynomial hierarchy collapses to the third level. As we explain below, we reach the same conclusion (see Theorem 17 in Section 4) without any assumption on the ...
... according to the same distribution (that depends only on the length of the input), then the existence of such a reduction implies that the polynomial hierarchy collapses to the third level. As we explain below, we reach the same conclusion (see Theorem 17 in Section 4) without any assumption on the ...
Every Property of Hyperfinite Graphs is Testable
... about the possibility of being planar (or not having any minor from a set of forbidden ones). They show that any graph come isomorphic. We will refer to such algorithms as property testing algorithms or testers, for short. See exact definitions in Section 2 ...
... about the possibility of being planar (or not having any minor from a set of forbidden ones). They show that any graph come isomorphic. We will refer to such algorithms as property testing algorithms or testers, for short. See exact definitions in Section 2 ...
Brownian Motion and Poisson Process
... Theorem 1. Normally distributed increments of Brownian motion If W (t) is a Brownian motion, then W (t) − W (0) is a normal random variable with mean µt and variance σ 2t, where µ and σ are constant real numbers. As a result of this theorem, we have the following density function of a Brownian ...
... Theorem 1. Normally distributed increments of Brownian motion If W (t) is a Brownian motion, then W (t) − W (0) is a normal random variable with mean µt and variance σ 2t, where µ and σ are constant real numbers. As a result of this theorem, we have the following density function of a Brownian ...
The Enigma Of Probability - Center for Cognition and Neuroethics
... The Finite World Argument To ban everything that contains an unlimited number of entities from the realm of the possible, as this argument does, is both too drastic and too feeble at the same time. It is too drastic because if implemented universally all of mathematics as we know it would break down ...
... The Finite World Argument To ban everything that contains an unlimited number of entities from the realm of the possible, as this argument does, is both too drastic and too feeble at the same time. It is too drastic because if implemented universally all of mathematics as we know it would break down ...
Randomness

Randomness is the lack of pattern or predictability in events. A random sequence of events, symbols or steps has no order and does not follow an intelligible pattern or combination. Individual random events are by definition unpredictable, but in many cases the frequency of different outcomes over a large number of events (or ""trials"") is predictable. For example, when throwing two dice, the outcome of any particular roll is unpredictable, but a sum of 7 will occur twice as often as 4. In this view, randomness is a measure of uncertainty of an outcome, rather than haphazardness, and applies to concepts of chance, probability, and information entropy.The fields of mathematics, probability, and statistics use formal definitions of randomness. In statistics, a random variable is an assignment of a numerical value to each possible outcome of an event space. This association facilitates the identification and the calculation of probabilities of the events. Random variables can appear in random sequences. A random process is a sequence of random variables whose outcomes do not follow a deterministic pattern, but follow an evolution described by probability distributions. These and other constructs are extremely useful in probability theory and the various applications of randomness.Randomness is most often used in statistics to signify well-defined statistical properties. Monte Carlo methods, which rely on random input (such as from random number generators or pseudorandom number generators), are important techniques in science, as, for instance, in computational science. By analogy, quasi-Monte Carlo methods use quasirandom number generators.Random selection is a method of selecting items (often called units) from a population where the probability of choosing a specific item is the proportion of those items in the population. For example, with a bowl containing just 10 red marbles and 90 blue marbles, a random selection mechanism would choose a red marble with probability 1/10. Note that a random selection mechanism that selected 10 marbles from this bowl would not necessarily result in 1 red and 9 blue. In situations where a population consists of items that are distinguishable, a random selection mechanism requires equal probabilities for any item to be chosen. That is, if the selection process is such that each member of a population, of say research subjects, has the same probability of being chosen then we can say the selection process is random.