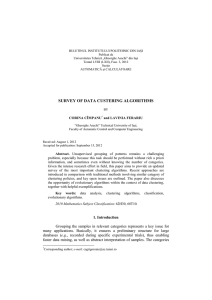
Ensemble of Feature Selection Techniques for High
... model, it suggests that the classification model has the highest probability for making a correct decision. It has also been shown that AUC has lower variance and is more reliable than other performance metrics such as precision, recall and F-measure. Using a single feature ranking technique may gen ...
... model, it suggests that the classification model has the highest probability for making a correct decision. It has also been shown that AUC has lower variance and is more reliable than other performance metrics such as precision, recall and F-measure. Using a single feature ranking technique may gen ...
Extracting Temporal Patterns from Interval-Based Sequences
... All the curves presented in the sequel were obtained by averaging the results on several (from 5 to 10) different datasets generated from the same parameters. Where not stated otherwise, the following default parameter values were used: |D| = 100, rP = 0.4, tN = 0.2, fmin = 0.1 and = ∞. We used th ...
... All the curves presented in the sequel were obtained by averaging the results on several (from 5 to 10) different datasets generated from the same parameters. Where not stated otherwise, the following default parameter values were used: |D| = 100, rP = 0.4, tN = 0.2, fmin = 0.1 and = ∞. We used th ...
Expectation–maximization algorithm

In statistics, an expectation–maximization (EM) algorithm is an iterative method for finding maximum likelihood or maximum a posteriori (MAP) estimates of parameters in statistical models, where the model depends on unobserved latent variables. The EM iteration alternates between performing an expectation (E) step, which creates a function for the expectation of the log-likelihood evaluated using the current estimate for the parameters, and a maximization (M) step, which computes parameters maximizing the expected log-likelihood found on the E step. These parameter-estimates are then used to determine the distribution of the latent variables in the next E step.