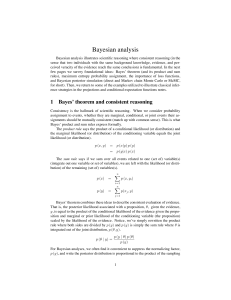
Bayesian analysis
... we take account of our background knowledge, , and evaluate the evidence in a manner consistent with both background knowledge and evidence. That is, the posterior likelihood (or distribution) is more aptly represented by p ( | y, ) p (y | , ) p ( | ) ...
... we take account of our background knowledge, , and evaluate the evidence in a manner consistent with both background knowledge and evidence. That is, the posterior likelihood (or distribution) is more aptly represented by p ( | y, ) p (y | , ) p ( | ) ...
BAYESIAN STATISTICS
... J. M. Bernardo. Bayesian Statistics Unlike most other branches of mathematics, conventional methods of statistical inference suffer from the lack of an axiomatic basis; as a consequence, their proposed desiderata are often mutually incompatible, and the analysis of the same data may well lead to in ...
... J. M. Bernardo. Bayesian Statistics Unlike most other branches of mathematics, conventional methods of statistical inference suffer from the lack of an axiomatic basis; as a consequence, their proposed desiderata are often mutually incompatible, and the analysis of the same data may well lead to in ...
Prior Elicitation from Expert Opinion
... It will not always be the case that we will have sufficient data to be able to ignore prior knowledge, and one example of this would be in the uncertainty in computer models application or modeling extreme events. Uncertain model input parameters are often assigned probability distributions entirely ...
... It will not always be the case that we will have sufficient data to be able to ignore prior knowledge, and one example of this would be in the uncertainty in computer models application or modeling extreme events. Uncertain model input parameters are often assigned probability distributions entirely ...
Bayesian Inference and Data Analysis
... the phylogenetics community for these reasons; a number of applications allow many demographic and evolutionary parameters to be estimated simultaneously. In the areas of population genetics and dynamical systems theory, approximate Bayesian computation (ABC) is also becoming increasingly popular. ...
... the phylogenetics community for these reasons; a number of applications allow many demographic and evolutionary parameters to be estimated simultaneously. In the areas of population genetics and dynamical systems theory, approximate Bayesian computation (ABC) is also becoming increasingly popular. ...
A primer in Bayesian Inference
... well documented free version of WINBUGS, suited for not too big models can be downloaded. The orientation was primarily medical statistics, but many ...
... well documented free version of WINBUGS, suited for not too big models can be downloaded. The orientation was primarily medical statistics, but many ...
Prior vs Likelihood vs Posterior Posterior Predictive Distribution
... 1. Simulate θi from θ|y; i = 1, . . . , m 2. Simulate ỹi from ỹ|θi (= ỹ|θi, y); i = 1, . . . , m The pairs (θi, ỹi) are draws from the joint distribution θ, ỹ|y. Therefore the ỹi are draws from ỹ|y. ...
... 1. Simulate θi from θ|y; i = 1, . . . , m 2. Simulate ỹi from ỹ|θi (= ỹ|θi, y); i = 1, . . . , m The pairs (θi, ỹi) are draws from the joint distribution θ, ỹ|y. Therefore the ỹi are draws from ỹ|y. ...
Objective Bayesian Statistics An Introduction to José M. Bernardo
... independent of the sample size, often exists. This is case whenever the statistical model belongs to the generalized exponential family, which includes many of the more frequently used statistical models. In contrast to frequentist statistics, Bayesian methods are independent on the possible existen ...
... independent of the sample size, often exists. This is case whenever the statistical model belongs to the generalized exponential family, which includes many of the more frequently used statistical models. In contrast to frequentist statistics, Bayesian methods are independent on the possible existen ...
An Introduction to Bayesian Statistics Without Using Equations
... no new inference should be made on the parameters whose posterior distributions are very similar to the prior distributions. Perhaps, those parameters should not be in the model to begin with. Some things to consider about the prior distributions are: (1) Are the limits of each parameter appropriate ...
... no new inference should be made on the parameters whose posterior distributions are very similar to the prior distributions. Perhaps, those parameters should not be in the model to begin with. Some things to consider about the prior distributions are: (1) Are the limits of each parameter appropriate ...
An Introduction to MCMC methods and Bayesian
... probability model’ However from a Bayesian point of view : • is unknown so should have a probability distribution reflecting our uncertainty about it before seeing the data • Therefore we specify a prior distribution p(θ) Note this is like the prevalence in the example ...
... probability model’ However from a Bayesian point of view : • is unknown so should have a probability distribution reflecting our uncertainty about it before seeing the data • Therefore we specify a prior distribution p(θ) Note this is like the prevalence in the example ...
A BAYESIAN MATHEMATICAL STATISTICS PRIMER Jos´ e M. Bernardo Universitat de Val`
... the many problems and limitations of the frequentist paradigm later discovered. Hard core statistical journals carry today a sizeable proportion of Bayesian papers (indeed a recent survey of Bayesian papers indexed in the Scientific Citation Index shows an exponential growth), but this does not yet ...
... the many problems and limitations of the frequentist paradigm later discovered. Hard core statistical journals carry today a sizeable proportion of Bayesian papers (indeed a recent survey of Bayesian papers indexed in the Scientific Citation Index shows an exponential growth), but this does not yet ...
Lecture 2 - eis.bris.ac.uk
... in many cases they are acceptable provided they result in a proper posterior distribution. • Uniform priors are often used as non-informative priors however it is worth noting that a uniform prior on one scale can be very informative on another. • For example: If we have an unknown variance we may p ...
... in many cases they are acceptable provided they result in a proper posterior distribution. • Uniform priors are often used as non-informative priors however it is worth noting that a uniform prior on one scale can be very informative on another. • For example: If we have an unknown variance we may p ...
bsyextra
... buried in Bunhill Cemetary, Moongate, London famous paper in 1763 Phil Trans Roy Soc London was Bayes the first with this idea? (Laplace?) ...
... buried in Bunhill Cemetary, Moongate, London famous paper in 1763 Phil Trans Roy Soc London was Bayes the first with this idea? (Laplace?) ...
DOCX - Economic Geography
... The term P(HA) is the prior probability distribution, often simply referred to as the prior. This is a distribution that characterizes our understanding of the phenomenon being modeled before we look at our data. The prior can be obtained from previous studies published in the literature, a pilot st ...
... The term P(HA) is the prior probability distribution, often simply referred to as the prior. This is a distribution that characterizes our understanding of the phenomenon being modeled before we look at our data. The prior can be obtained from previous studies published in the literature, a pilot st ...
Bayesian Statistics 3 Normal Data
... Compared to the inference machinery of classical statistics, with its p-values, confidence intervals, significance levels, bias, etc., Bayesian inference is straightforward: the inference result is the posterior, which is a probability distribution. Various descriptive statistical tools are availabl ...
... Compared to the inference machinery of classical statistics, with its p-values, confidence intervals, significance levels, bias, etc., Bayesian inference is straightforward: the inference result is the posterior, which is a probability distribution. Various descriptive statistical tools are availabl ...
2041-2223-2-24-S1
... method does not require a "burn-in" period [80] and tests whether it is possible to recover the MCRA of the sample with the number of performed generations. The basic demographic characteristics implemented in this forward simulator are: (i) diploidy of individuals, (ii) equal proportions of the two ...
... method does not require a "burn-in" period [80] and tests whether it is possible to recover the MCRA of the sample with the number of performed generations. The basic demographic characteristics implemented in this forward simulator are: (i) diploidy of individuals, (ii) equal proportions of the two ...
Infinite Exchangeability and Random Set Partitions
... Instead of constructing statistics for comparing variety means in pairs, we start with an infinitely exchangeable Gaussian prior for the variety effects such that each pair of varieties is equal with positive prior probability. Consistency conditions on joint exchangeable priors involving set partit ...
... Instead of constructing statistics for comparing variety means in pairs, we start with an infinitely exchangeable Gaussian prior for the variety effects such that each pair of varieties is equal with positive prior probability. Consistency conditions on joint exchangeable priors involving set partit ...
A Poisson Point Process Model with Its Applications and Network Analysis of Genome-wide Association Study
... procedures from improper priors. However, improper priors may also lead to Bayes procedures that are paradoxical or otherwise unsatisfactory prompting some authors to insist that all priors be proper. Our model begins with the observation that an improper measure on the parameter space satisfying Ki ...
... procedures from improper priors. However, improper priors may also lead to Bayes procedures that are paradoxical or otherwise unsatisfactory prompting some authors to insist that all priors be proper. Our model begins with the observation that an improper measure on the parameter space satisfying Ki ...
Objective Bayes and Conditional Probability
... In Bayesian parametric inference, in the absence of subjective prior information about the parameter of interest, it is natural to consider use of an objective prior which leads to posterior probability quantiles which have, at least to some higher order approximation in terms of the sample size, th ...
... In Bayesian parametric inference, in the absence of subjective prior information about the parameter of interest, it is natural to consider use of an objective prior which leads to posterior probability quantiles which have, at least to some higher order approximation in terms of the sample size, th ...
Posterior Distributions on Parameter Space via Group Invariance
... In answering the question “what is the probability distribution of the parameter given observed data” when there is little or no prior knowledge on the parameter values, one may consider three types of statistical inference: Bayesian, frequentist, and group invariance-based. The focus here is on the ...
... In answering the question “what is the probability distribution of the parameter given observed data” when there is little or no prior knowledge on the parameter values, one may consider three types of statistical inference: Bayesian, frequentist, and group invariance-based. The focus here is on the ...