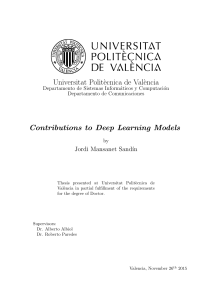
cG Sufatrio, Darell JJ Tan, Tong-Wei Chua, Vrizlynn LL
... and easier comparisons among them. Additionally, we update Enck [2011] by covering many later works between 2011 and 2014 in the fast-growing Android security field. In their comprehensive survey work, Zhou and Jiang [2012] characterized 1,260 malware samples belonging to 49 different families, whic ...
... and easier comparisons among them. Additionally, we update Enck [2011] by covering many later works between 2011 and 2014 in the fast-growing Android security field. In their comprehensive survey work, Zhou and Jiang [2012] characterized 1,260 malware samples belonging to 49 different families, whic ...
artificial intelligence (luger, 6th, 2008)
... belief networks, and related graphical models. Our expansion includes greater use of probabilistic finite state machines, hidden Markov models, and dynamic programming with the Earley parser and implementing the Viterbi algorithm. Other topics, such as emergent computation, ontologies, stochastic pa ...
... belief networks, and related graphical models. Our expansion includes greater use of probabilistic finite state machines, hidden Markov models, and dynamic programming with the Earley parser and implementing the Viterbi algorithm. Other topics, such as emergent computation, ontologies, stochastic pa ...
Deloitte`s point of view on IBM Watson
... Cognitive computing is starting to emerge as a real opportunity and threat for many businesses. It is a component of the broader trend around big data, but it is particularly important because cognitive computing focuses on the realm of unstructured data, which is clearly dominant in volume over tha ...
... Cognitive computing is starting to emerge as a real opportunity and threat for many businesses. It is a component of the broader trend around big data, but it is particularly important because cognitive computing focuses on the realm of unstructured data, which is clearly dominant in volume over tha ...
Contents | Zoom in | Zoom out Search Issue | Next Page For
... include human-computer interaction, optimization in large-scale systems, naturedinspired computing, Internet-of-Things, etc. For computational intelligence to become an integral component in new technological enterprises, it is crucial that graduating engineers and computer scientists are familiar w ...
... include human-computer interaction, optimization in large-scale systems, naturedinspired computing, Internet-of-Things, etc. For computational intelligence to become an integral component in new technological enterprises, it is crucial that graduating engineers and computer scientists are familiar w ...
Survey of Applications Integrating Constraint Satisfaction and Case
... 3.2 CBR and its Application to Real -World Problems Case-based reasoning is seeing its application to more and more real-world problems. The reasons that CBR has real-world usefulness and application are many. The main benefits that CBR can provide as a reasoning technique include: easing knowledge ...
... 3.2 CBR and its Application to Real -World Problems Case-based reasoning is seeing its application to more and more real-world problems. The reasons that CBR has real-world usefulness and application are many. The main benefits that CBR can provide as a reasoning technique include: easing knowledge ...
Time series

A time series is a sequence of data points, typically consisting of successive measurements made over a time interval. Examples of time series are ocean tides, counts of sunspots, and the daily closing value of the Dow Jones Industrial Average. Time series are very frequently plotted via line charts. Time series are used in statistics, signal processing, pattern recognition, econometrics, mathematical finance, weather forecasting, intelligent transport and trajectory forecasting, earthquake prediction, electroencephalography, control engineering, astronomy, communications engineering, and largely in any domain of applied science and engineering which involves temporal measurements.Time series analysis comprises methods for analyzing time series data in order to extract meaningful statistics and other characteristics of the data. Time series forecasting is the use of a model to predict future values based on previously observed values. While regression analysis is often employed in such a way as to test theories that the current values of one or more independent time series affect the current value of another time series, this type of analysis of time series is not called ""time series analysis"", which focuses on comparing values of a single time series or multiple dependent time series at different points in time.Time series data have a natural temporal ordering. This makes time series analysis distinct from cross-sectional studies, in which there is no natural ordering of the observations (e.g. explaining people's wages by reference to their respective education levels, where the individuals' data could be entered in any order). Time series analysis is also distinct from spatial data analysis where the observations typically relate to geographical locations (e.g. accounting for house prices by the location as well as the intrinsic characteristics of the houses). A stochastic model for a time series will generally reflect the fact that observations close together in time will be more closely related than observations further apart. In addition, time series models will often make use of the natural one-way ordering of time so that values for a given period will be expressed as deriving in some way from past values, rather than from future values (see time reversibility.)Time series analysis can be applied to real-valued, continuous data, discrete numeric data, or discrete symbolic data (i.e. sequences of characters, such as letters and words in the English language.).