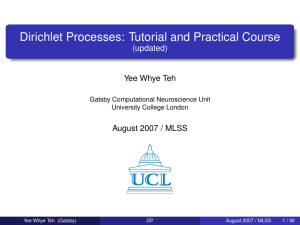
Dirichlet Processes: Tutorial and Practical Course (updated)
... Suppose G ∼ DP(α, H). G is a (random) probability measure over X. We can treat it as a distribution over X. Let θ1 , . . . , θ n ∼ G be a random variable with distribution G. We saw in the demo that draws from a Dirichlet ...
... Suppose G ∼ DP(α, H). G is a (random) probability measure over X. We can treat it as a distribution over X. Let θ1 , . . . , θ n ∼ G be a random variable with distribution G. We saw in the demo that draws from a Dirichlet ...
A Tutorial on Dirichlet Processes and Hierarchical Dirichlet Processes
... Draw θ1 , . . . , θn from a Pòlya’s urn scheme. They take on K < n distinct values, say θ1∗ , . . . , θK∗ . This defines a partition of 1, . . . , n into K clusters, such that if i is in cluster k , then θi = θk∗ . Random draws θ1 , . . . , θn from a Pòlya’s urn scheme induces a random partition of ...
... Draw θ1 , . . . , θn from a Pòlya’s urn scheme. They take on K < n distinct values, say θ1∗ , . . . , θK∗ . This defines a partition of 1, . . . , n into K clusters, such that if i is in cluster k , then θi = θk∗ . Random draws θ1 , . . . , θn from a Pòlya’s urn scheme induces a random partition of ...
Introduction to the Dirichlet Distribution and Related
... the variability of these pmfs. Different Dirichlet distributions can be used to model documents by different authors or documents on different topics. In this section, we describe the Dirichlet distribution and some of its properties. In Sections 1.2 and 1.4, we illustrate common modeling scenarios ...
... the variability of these pmfs. Different Dirichlet distributions can be used to model documents by different authors or documents on different topics. In this section, we describe the Dirichlet distribution and some of its properties. In Sections 1.2 and 1.4, we illustrate common modeling scenarios ...
Distributional properties of means of random probability measures
... reduces to studying the random quantity R x P̃(dx) given that R |x| P̃(dx) < ∞ almost surely. In particular, in [9] the authors introduce a series of tools and techniques that, later in [10], turned R out to be fundamental for the determination of the probability distribution of X f (x)P̃(dx) when P ...
... reduces to studying the random quantity R x P̃(dx) given that R |x| P̃(dx) < ∞ almost surely. In particular, in [9] the authors introduce a series of tools and techniques that, later in [10], turned R out to be fundamental for the determination of the probability distribution of X f (x)P̃(dx) when P ...
Dirichlet mixtures - Center for Bioinformatics and Computational
... with identical parameters, except that α′' = α' + 1. ...
... with identical parameters, except that α′' = α' + 1. ...
Slides - RAD Lab - University of California, Berkeley
... • A general way to obtain distributions on countably infinite spaces • The classical example: Define an infinite sequence of beta random variables: • And then define an infinite random sequence as follows: ...
... • A general way to obtain distributions on countably infinite spaces • The classical example: Define an infinite sequence of beta random variables: • And then define an infinite random sequence as follows: ...
Dirichlet Processes
... H, is a normal distribution with zero mean and standard deviation 50. (B) The base measure, H, is a normal distribution with zero mean and standard deviation 20. The base measure is shown by the solid black lines in each plot. Different columns correspond to different concentration parameters. Note ...
... H, is a normal distribution with zero mean and standard deviation 50. (B) The base measure, H, is a normal distribution with zero mean and standard deviation 20. The base measure is shown by the solid black lines in each plot. Different columns correspond to different concentration parameters. Note ...
Estimating probabilities from counts with a prior of uncertain reliability
... distribution, P (A) = λ exp(−λA), with mean 1/λ, might be appropriate. Fortunately, we can often avoid selecting an explicit hyperprior. In practice, given sufficient data, the probability of that data P (n|A) is a smooth, sharply peaked function of A. This is illustrated in figure 1 using 107 obser ...
... distribution, P (A) = λ exp(−λA), with mean 1/λ, might be appropriate. Fortunately, we can often avoid selecting an explicit hyperprior. In practice, given sufficient data, the probability of that data P (n|A) is a smooth, sharply peaked function of A. This is illustrated in figure 1 using 107 obser ...