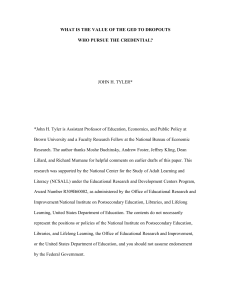
The CALIS Procedure
... fixed levels. For maximum likelihood (default) and generalized least squares estimation in PROC CALIS, the random variables are assumed to have an approximately multivariate normal distribution. Nonnormality, especially high kurtosis, can produce poor estimates and grossly incorrect standard errors ...
... fixed levels. For maximum likelihood (default) and generalized least squares estimation in PROC CALIS, the random variables are assumed to have an approximately multivariate normal distribution. Nonnormality, especially high kurtosis, can produce poor estimates and grossly incorrect standard errors ...
When Everyone Misses on the Same Side: Debiased Earnings Surprises and Stock Returns
... Essentially, when some of the forecasts are biased enough, it is better to discard magnitudes and to simply count the fraction of misses. If everyone misses on the same side, we know that even unbiased forecasts missed on the same side as biased forecasts, which is enough to know that the market is ...
... Essentially, when some of the forecasts are biased enough, it is better to discard magnitudes and to simply count the fraction of misses. If everyone misses on the same side, we know that even unbiased forecasts missed on the same side as biased forecasts, which is enough to know that the market is ...
Testing Conditional Factor Models
... bandwidths allows us to better adjust the estimators for these effects. However, in order to avoid cumbersome notation, we present the asymptotic results for the estimators α̂τ and β̂τ assuming one common bandwidth, h, across all stocks. The asymptotic results are identical in the case with bandwidt ...
... bandwidths allows us to better adjust the estimators for these effects. However, in order to avoid cumbersome notation, we present the asymptotic results for the estimators α̂τ and β̂τ assuming one common bandwidth, h, across all stocks. The asymptotic results are identical in the case with bandwidt ...
SPATIAL DATA ANALYSIS WITH GIS: AN INTRODUCTION TO
... analysis of spatial data. I return to this issue in more detail below. The crucial role of location for spatial data, both in an absolute sense (coordinates) and in a relative sense (spatial arrangement, distance) has major implications for the way in which they should be treated in statistical anal ...
... analysis of spatial data. I return to this issue in more detail below. The crucial role of location for spatial data, both in an absolute sense (coordinates) and in a relative sense (spatial arrangement, distance) has major implications for the way in which they should be treated in statistical anal ...
Vector Autoregressions with Parsimoniously Time Varying
... the path of the parameter vector in a non parametric way. In this paper we assume the probability αT for an increment to be different from zero to depend on the sample length T , specifically αT = k α T −a , where k α and a are positive constants. In the case of a single variable this leads to an e ...
... the path of the parameter vector in a non parametric way. In this paper we assume the probability αT for an increment to be different from zero to depend on the sample length T , specifically αT = k α T −a , where k α and a are positive constants. In the case of a single variable this leads to an e ...
Diagnosing Harmful Collinearity in Moderated Regressions
... where U and V are ratio scaled explanatory variables in N-dimensional data vectors.2 Because the interaction term UV shares information with both U and V, there may be correlations and/or collinearity between these variables. Correlations refer to linear co-variability of two variables around their ...
... where U and V are ratio scaled explanatory variables in N-dimensional data vectors.2 Because the interaction term UV shares information with both U and V, there may be correlations and/or collinearity between these variables. Correlations refer to linear co-variability of two variables around their ...
Teradata Warehouse Miner User Guide
... The information contained in this document may contain references or cross-references to features, functions, products, or services that are not announced or available in your country. Such references do not imply that Teradata Corporation intends to announce such features, functions, products, or s ...
... The information contained in this document may contain references or cross-references to features, functions, products, or services that are not announced or available in your country. Such references do not imply that Teradata Corporation intends to announce such features, functions, products, or s ...
Theano: A CPU and GPU Math Compiler in Python
... differentiable loss function which is the expectation of some per-example loss over a set of training examples. SGD estimates this expectation with an average over one or several examples and performs a step in the approximate direction of steepest descent. Though more sophisticated algorithms for n ...
... differentiable loss function which is the expectation of some per-example loss over a set of training examples. SGD estimates this expectation with an average over one or several examples and performs a step in the approximate direction of steepest descent. Though more sophisticated algorithms for n ...
Multiple Fixed Effects in Nonlinear Panel Data Models - Theory and Evidence
... However, econometric theory has mostly focused on single fixed effects. The present paper attempts to bridge part of this gap by looking at some specific nonlinear models. The empirical relevance is demonstrated using Monte Carlo simulations and an application to international trade data. This paper ...
... However, econometric theory has mostly focused on single fixed effects. The present paper attempts to bridge part of this gap by looking at some specific nonlinear models. The empirical relevance is demonstrated using Monte Carlo simulations and an application to international trade data. This paper ...
CDS Exposure and Credit Spreads
... obligation if a credit event occurs. In return for protection, the CDS buyer makes fixed payments to the protection seller generally in quarterly installments. The annual fee for the protection is called the CDS spread. The protection seller (counterparty) receives the spread and pays out the losses ...
... obligation if a credit event occurs. In return for protection, the CDS buyer makes fixed payments to the protection seller generally in quarterly installments. The annual fee for the protection is called the CDS spread. The protection seller (counterparty) receives the spread and pays out the losses ...
Linear regression
In statistics, linear regression is an approach for modeling the relationship between a scalar dependent variable y and one or more explanatory variables (or independent variables) denoted X. The case of one explanatory variable is called simple linear regression. For more than one explanatory variable, the process is called multiple linear regression. (This term should be distinguished from multivariate linear regression, where multiple correlated dependent variables are predicted, rather than a single scalar variable.)In linear regression, data are modeled using linear predictor functions, and unknown model parameters are estimated from the data. Such models are called linear models. Most commonly, linear regression refers to a model in which the conditional mean of y given the value of X is an affine function of X. Less commonly, linear regression could refer to a model in which the median, or some other quantile of the conditional distribution of y given X is expressed as a linear function of X. Like all forms of regression analysis, linear regression focuses on the conditional probability distribution of y given X, rather than on the joint probability distribution of y and X, which is the domain of multivariate analysis.Linear regression was the first type of regression analysis to be studied rigorously, and to be used extensively in practical applications. This is because models which depend linearly on their unknown parameters are easier to fit than models which are non-linearly related to their parameters and because the statistical properties of the resulting estimators are easier to determine.Linear regression has many practical uses. Most applications fall into one of the following two broad categories: If the goal is prediction, or forecasting, or error reduction, linear regression can be used to fit a predictive model to an observed data set of y and X values. After developing such a model, if an additional value of X is then given without its accompanying value of y, the fitted model can be used to make a prediction of the value of y. Given a variable y and a number of variables X1, ..., Xp that may be related to y, linear regression analysis can be applied to quantify the strength of the relationship between y and the Xj, to assess which Xj may have no relationship with y at all, and to identify which subsets of the Xj contain redundant information about y.Linear regression models are often fitted using the least squares approach, but they may also be fitted in other ways, such as by minimizing the ""lack of fit"" in some other norm (as with least absolute deviations regression), or by minimizing a penalized version of the least squares loss function as in ridge regression (L2-norm penalty) and lasso (L1-norm penalty). Conversely, the least squares approach can be used to fit models that are not linear models. Thus, although the terms ""least squares"" and ""linear model"" are closely linked, they are not synonymous.